Application of AI for Accelerated Industrial Real Estate Due Diligence
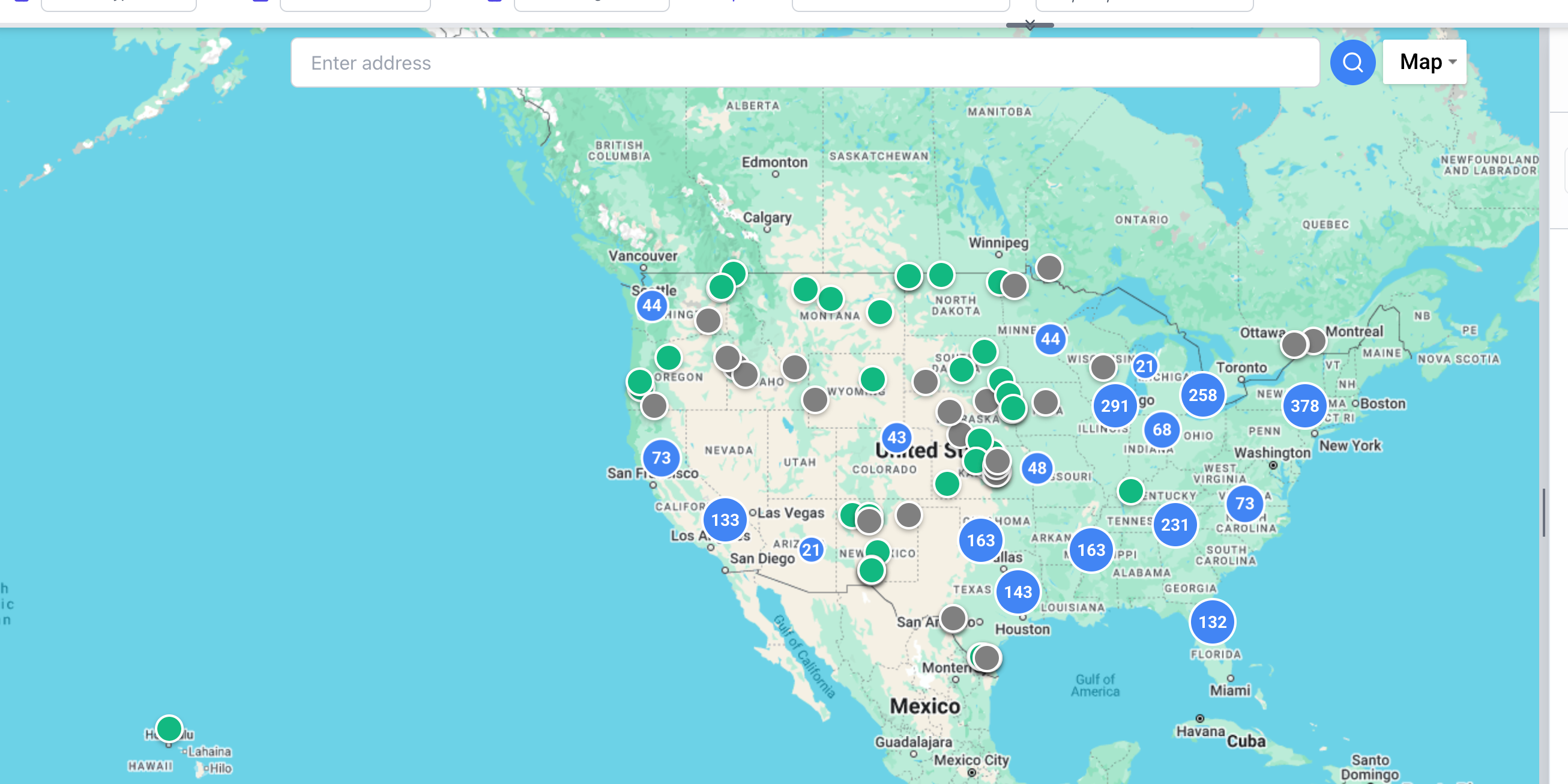
Executive Summary:
Traditional due diligence processes for industrial real estate, while essential, are characterized by significant time requirements, high resource allocation, and data latency. This report details how we, at AnalystAI, applied our platform to enable a major global real estate firm to automate and enhance its due diligence workflow.
Our platform reduced a typical 30-day analysis cycle to 3 days, decreased associated costs substantially, expanded data coverage, and introduced dynamic data refresh capabilities. Key outputs we provided included both static, citation-backed PDF reports suitable for investment committee review and interactive dashboards for granular market exploration.
1. Context: Conventional Due Diligence Methodologies and Constraints
Standard due diligence protocols for evaluating industrial real estate opportunities typically involve extensive manual data collection and analysis by specialized teams. Core activities include:
- Manual Data Aggregation: Analysts manually gather information from diverse, often unstructured sources such as government land registries, zoning portals, municipal planning documents, corporate websites, news outlets, and property listings. This process is inherently time-consuming.
- Data Integration Challenges: Information regarding population dynamics, competitor facility specifications (e.g., Gross Floor Area (GFA), clear height), infrastructure availability, and local economic activity (e.g., manufacturer expansions, capital expenditures) is often siloed. Synthesizing these disparate datasets to form a cohesive market view requires significant analytical effort and presents risks of error or oversight.
- Time and Cost Intensity: A comprehensive due diligence exercise for a specific market or portfolio can typically require approximately four weeks of dedicated analyst time, translating to considerable personnel costs, often reaching six-figure sums for complex engagements.
- Data Latency and Static Reporting: The output is frequently a static report representing market conditions at a specific point in time. The inherent delay in production means decisions may be based on data that is weeks or months old, a significant constraint in dynamic markets.
[Figure 1: Conceptual diagram illustrating traditional, multi-source manual data gathering feeding into siloed analyses.]
2. Analyst AI Platform: How We Automate Data Aggregation and Analysis
To address these constraints, the client firm deployed our AnalystAI platform. Our system automates key stages of the due diligence data workflow:
- Systematic Data Pipeline: We execute an automated sequence:
- Data Extraction: Programmatic scraping and ingestion of data from pre-defined public and licensed sources relevant to industrial real estate.
- Data Cleansing & Structuring: Normalization and organization of varied data formats into a consistent schema.
- Data Enrichment: Augmentation of core data with related information (e.g., linking property data to tenant information or local economic indicators).
- Validation & Verification: Cross-referencing data points against multiple sources and applying validation rules (e.g., using Pydantic models) to ensure data integrity.
- Output Generation: Compiling validated data and derived insights into specified output formats.
- Targeted Data Domains: We configured our platform to capture and analyze data pertinent to industrial real estate evaluation, including:
- Demographic and Economic Data (Census, BLS, local economic reports).
- Existing and Planned Facility Specifications (Size, height, age, tenancy, ownership).
- Competitor and Ecosystem Activity (New developments, expansions, major investments from news and planning sources).
- Logistics Infrastructure (Proximity to highways, ports, rail).
- Underlying Technology: Our platform utilizes specific AI components developed by AnalystAI:
- Optical Character Recognition (OCR) & Vision Models: We leverage our own fine-tuned OCR and vision models for extracting structured data from image-based documents (e.g., PDF site plans, spec sheets).
- Natural Language Processing (NLP) & Retrieval-Augmented Generation (RAG): For identifying and synthesizing relevant information from unstructured text sources (e.g., news articles, planning commission minutes).
- Citation Engine: Critically, our system tracks and provides source citations for verifiable data points, maintaining transparency and auditability – a core principle of our approach. Additionally, we use our own curated dataset of real estate transactions and locations, created by our AI agents and enriched by our domain expertise, to enhance the accuracy and comprehensiveness of our analysis.
3. Output Formats and Data Presentation We Delivered
Our platform generated two primary outputs tailored to the client's workflow:
- Static PDF Report: A comprehensive document including an executive summary, thematic maps (e.g., heat maps of industrial density), data tables, and a detailed appendix. All key metrics and qualitative statements derived from external sources included verifiable citations. We aligned the report format with the client's established templates for investment committee submissions.
- Dynamic Interactive Dashboard: A web-based interface we provided, allowing users to:
- Geospatially Explore Data: Navigate a map interface displaying property locations, competitor sites, infrastructure, and demographic overlays.
- Filter and Query: Apply user-defined filters based on parameters such as facility size, construction date, tenant type, or proximity to logistics nodes.
- Access Granular Detail: Retrieve detailed information cards for specific properties or data points, often linking back to source documents or citations.
4. Quantifiable Performance Improvements Achieved
The deployment of our Analyst AI platform yielded measurable improvements over the traditional methodology:
- Reduced Analysis Cycle Time: The end-to-end process we facilitated, from data ingestion to report generation, was completed in 3 days, compared to the typical 30-day manual process (a 90% reduction).
- Cost Efficiency: Associated project costs were reduced by an estimated 70-80% compared to equivalent manual due diligence engagements, primarily due to the reduction in required analyst hours enabled by our automation.
- Increased Data Coverage: Our automated system identified approximately 2x the number of relevant facilities and incorporated data from 5x more news articles and public announcements within the target geography compared to prior manual efforts of similar duration.
- Data Refresh Capability: We configured the platform for automated data updates at a 90-day cadence, enabling ongoing market monitoring. On-demand refreshes were also made possible.
5. Implications of Dynamic Data Refresh Capabilities We Provide
The ability to automatically update the dataset and associated outputs, a key feature of our platform, provides distinct advantages:
- Continuous Market Monitoring: Enables near real-time awareness of significant market events (e.g., new large-scale lease signings, competitor development filings, major infrastructure project approvals).
- Proactive Risk/Opportunity Identification: Facilitates earlier detection of emerging trends or potential disruptions within the target market or supply chain ecosystem.
- Enhanced Decision Support: Ensures that ongoing asset management and future investment decisions are based on current, rather than lagging, market intelligence delivered by our system.
6. Strategic Implications for Real Estate Investment Firms Utilizing Our Technology
The observed outcomes suggest several strategic implications for large-scale real estate investors and advisors who partner with Analyst AI:
- Analyst Role Evolution: Our AI platform automates routine data collection and synthesis, allowing skilled analysts to concentrate on higher-value activities such as strategic interpretation, complex deal structuring, and client advisory.
- Improved Data Integrity and Trust: The systematic inclusion of verifiable citations, a cornerstone of our platform's design, addresses concerns regarding AI as a "black box," enhancing confidence in the data underpinning investment recommendations.
- Enhanced Communication: Interactive data visualizations, delivered through our dashboards, can improve the clarity and speed with which complex market analyses are communicated to internal committees and external stakeholders (e.g., Limited Partners).
- Potential for Cumulative Advantage: Firms adopting our technology may benefit from faster analysis cycles, broader data access, and potentially improved model accuracy over time as we enhance datasets and algorithms.
7. Potential Future Applications and Development Directions at Analyst AI
The technology underlying this application has potential extensions we are actively exploring within real estate investment and management, including:
- Integration with asset management systems for dynamic portfolio monitoring ("digital twins").
- Incorporation of alternative datasets (e.g., ESG metrics, climate risk data, granular logistics flows).
- Cross-asset class analysis to identify correlations and arbitrage opportunities (e.g., between logistics demand, data center development, and cold storage).
By applying our AnalystAI platform, real estate investors can now complete due diligence, a process that once took months, in mere days. This significant reduction in time, combined with expanded data coverage and dynamic updates, signifies a substantial shift from traditional methods. While human expertise remains essential for strategic decision-making, our AI-driven platform empowers investors with enhanced analytical capabilities and a competitive edge in the data-driven investment landscape. This transformation extends beyond real estate, promising to reshape the future of all investment analysis.