Improving Financial Models with AI
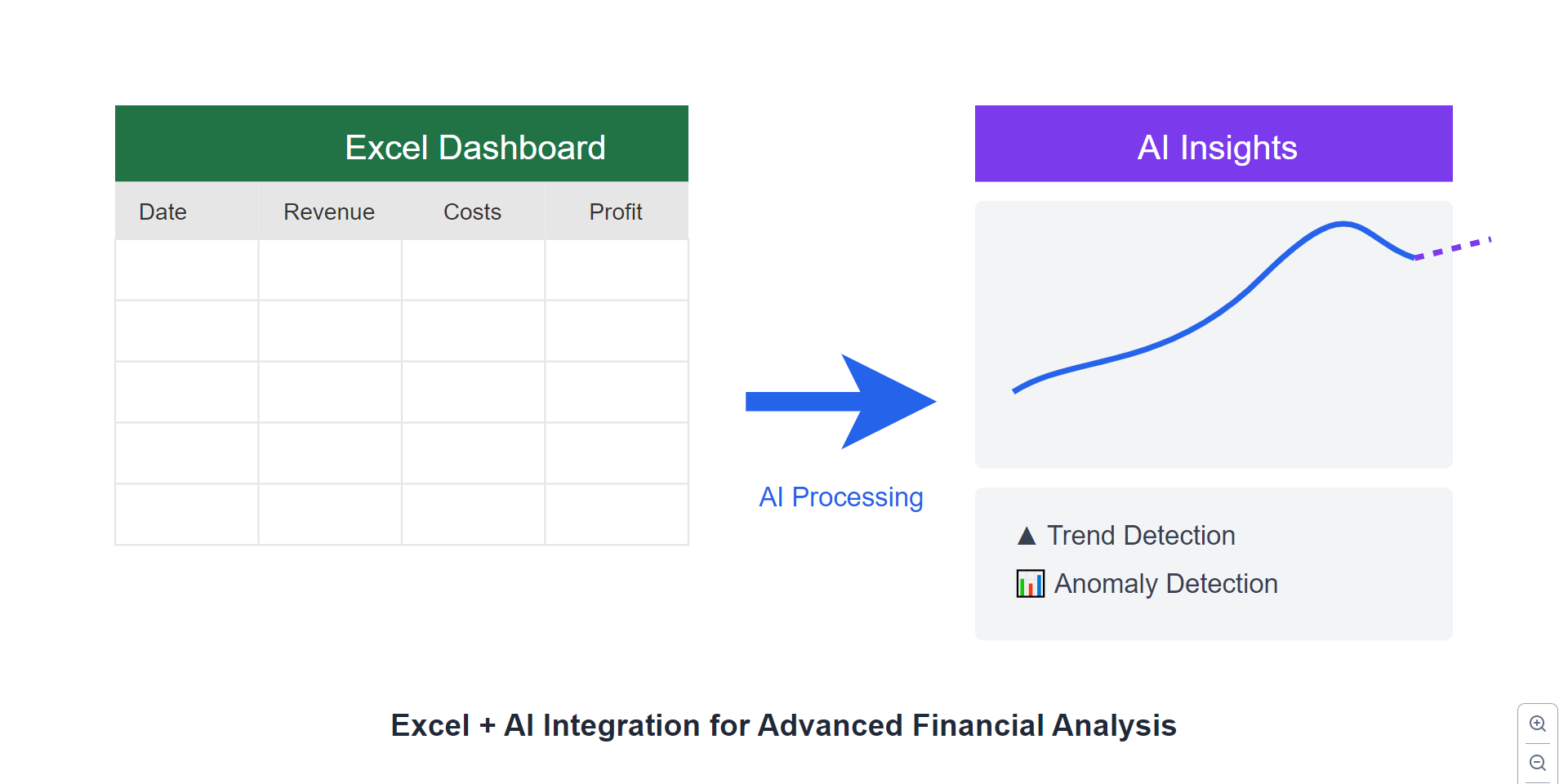
Improving Financial Models with AI: Bridging Excel and Advanced Insights
In finance, precision isn’t just desirable; it’s essential. While Excel has long been a mainstay for crafting financial models, advancing technologies and increasingly complex data needs are pushing traditional tools to their limits. Now, Artificial Intelligence (AI) offers a new horizon—one that doesn’t just streamline processes but fundamentally redefines decision-making in finance by enhancing accuracy, agility, and insights.
Contents Overview
- Why AI is Crucial for Financial Model Accuracy
- AI and Excel: Enhancing Traditional Financial Tools with AI
- The Role of Engineering in Financial AI Model Integration
- How AI Elevates Financial Insights Through Excel
- AI as a Financial Interpreter: The Importance of Interactivity in Financial Models
Why AI is Crucial for Financial Model Accuracy
The precision required in financial models is challenging for traditional tools, especially as data grows more complex and time-sensitive. Traditional Excel models are constrained by limitations in handling large datasets and providing real-time analysis. By integrating AI, financial analysts can go beyond traditional data manipulation to achieve real-time trend predictions, risk management, and enhanced strategy optimization.
According to LeewayHertz, AI enables more robust forecasting and scenario analysis by processing extensive datasets in real-time—a benefit that ensures accuracy and relevance, addressing gaps that static models often cannot fill.
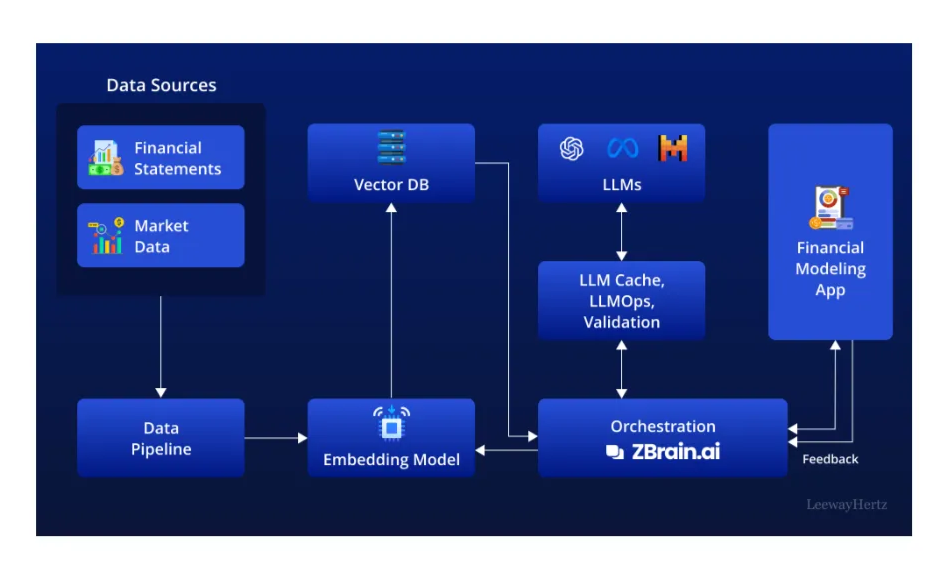
an illustration of financial models with data sources by Leeway Hertz
AI and Excel: Enhancing Traditional Financial Tools with AI
Excel remains a reliable foundation for many financial analysts, but it wasn’t designed for the dynamic, real-time insights required today. Adding AI into Excel workflows can solve common challenges by simplifying calculations, enabling predictive analytics, and adding enhanced data visualization capabilities. For example, AI automates data extraction and analysis, accelerating processes that typically demand significant manual work.
Excel becomes a far more potent tool for decision-making when paired with AI, allowing users to shift from mere data handling to sophisticated financial analysis.
The Role of Engineering in Financial AI Model Integration
Adopting AI in financial models isn’t plug-and-play; it requires custom engineering to ensure that AI solutions align precisely with an organization’s unique data requirements. Off-the-shelf AI tools are seldom suitable for financial applications, as they fail to capture the nuances of proprietary data, customized workflows, and regulatory obligations.
Engineering is critical for customizing AI algorithms to align with an institution’s financial models, goals, and data types. Without this tailored approach, the AI may yield inaccurate or irrelevant results, jeopardizing decision quality. The integration process demands an understanding of both finance and AI technology, bridging a gap often underestimated by generic AI solutions.
How AI Elevates Financial Insights Through Excel
The true power of AI-enhanced Excel lies in how it transforms data management into actionable insight creation. AI doesn’t just handle vast amounts of data; it interprets patterns and connections that might otherwise go unnoticed. This level of analysis enables financial analysts to make better investment decisions and manage risks proactively by recognizing and acting on subtle data correlations.
AI also allows for continuous model refinement, adjusting assumptions in real-time based on changing conditions. This adaptability means financial models become agile tools, staying relevant as market dynamics shift. The interaction between AI and financial models fosters a proactive, flexible approach, crucial for accurate financial planning.
AI as a Financial Interpreter: The Importance of Interactivity in Financial Models
One of AI’s most promising aspects is its ability to act as an interpreter for financial models. Traditional models often require expert interpretation, with analysts extracting insights from static data points. However, AI can add interpretive layers, generating contextual insights and explaining the reasoning behind the numbers.
For example, Talonic AI demonstrates how AI models can simulate scenarios to assess potential impacts of market shifts on financial outcomes. This capability is invaluable for stress testing and scenario planning, enhancing transparency around possible outcomes.
Conclusion
The future of financial modeling lies in the synergy between AI and traditional tools like Excel. By investing in tailored AI solutions that match an organization’s unique financial needs, businesses can transform their models from static resources into dynamic engines for insight and strategy. However, the key to unlocking this potential lies in thoughtful integration—prioritizing accuracy, regulatory compliance, and custom engineering.