Smaller AI Models Are Closing the Gap
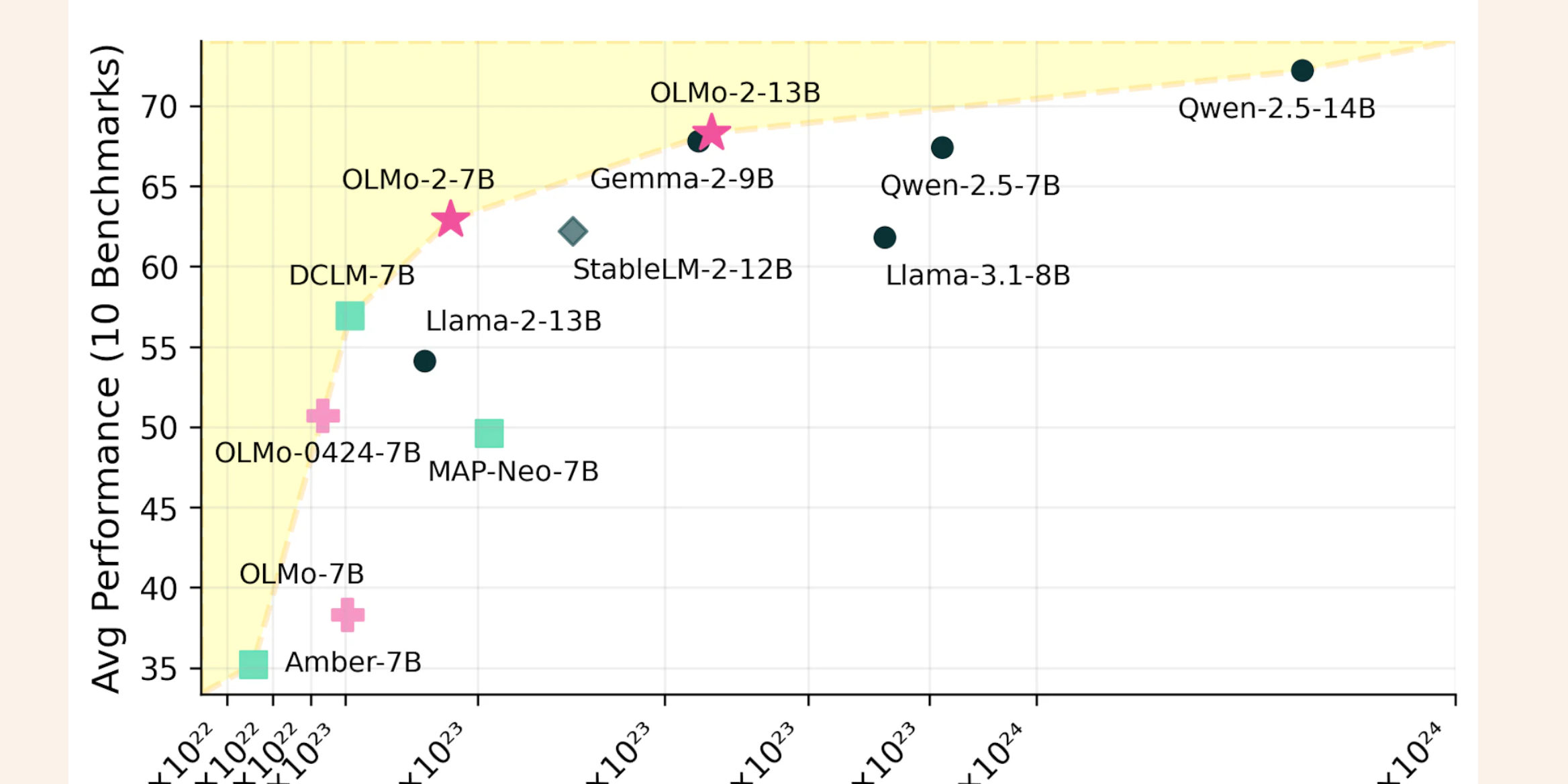
Smaller AI Models Are Closing the Gap
Smaller AI models are becoming more capable, as demonstrated by the latest OLMo-2-7B. The model performs significantly better than previous versions while requiring fewer resources. As seen in the chart, it outperforms many similarly sized models and approaches the performance of larger ones, like Llama-2-13B, in key benchmarks.
Implications for AI Development
Efficiency: Smaller models reduce computational requirements, enabling faster inference and lower operating costs.
Privacy: Open-source models like OLMo-2-7B can be deployed locally, allowing enterprises to maintain control over sensitive data while adopting AI solutions.
Open-Source Opportunity: High-performing smaller models create new opportunities for enterprises. They lower barriers to access, enabling businesses to build and deploy advanced AI solutions without relying on proprietary systems.
What’s Next
If these trends continue, smaller models could meet most enterprise needs, offering faster, cost-effective, and privacy-compliant AI solutions. For the open-source ecosystem, this means broader adoption and faster innovation, as businesses can now integrate advanced capabilities without the need for massive computational resources or external dependencies.