Using GraphRAG for High Quality Answers
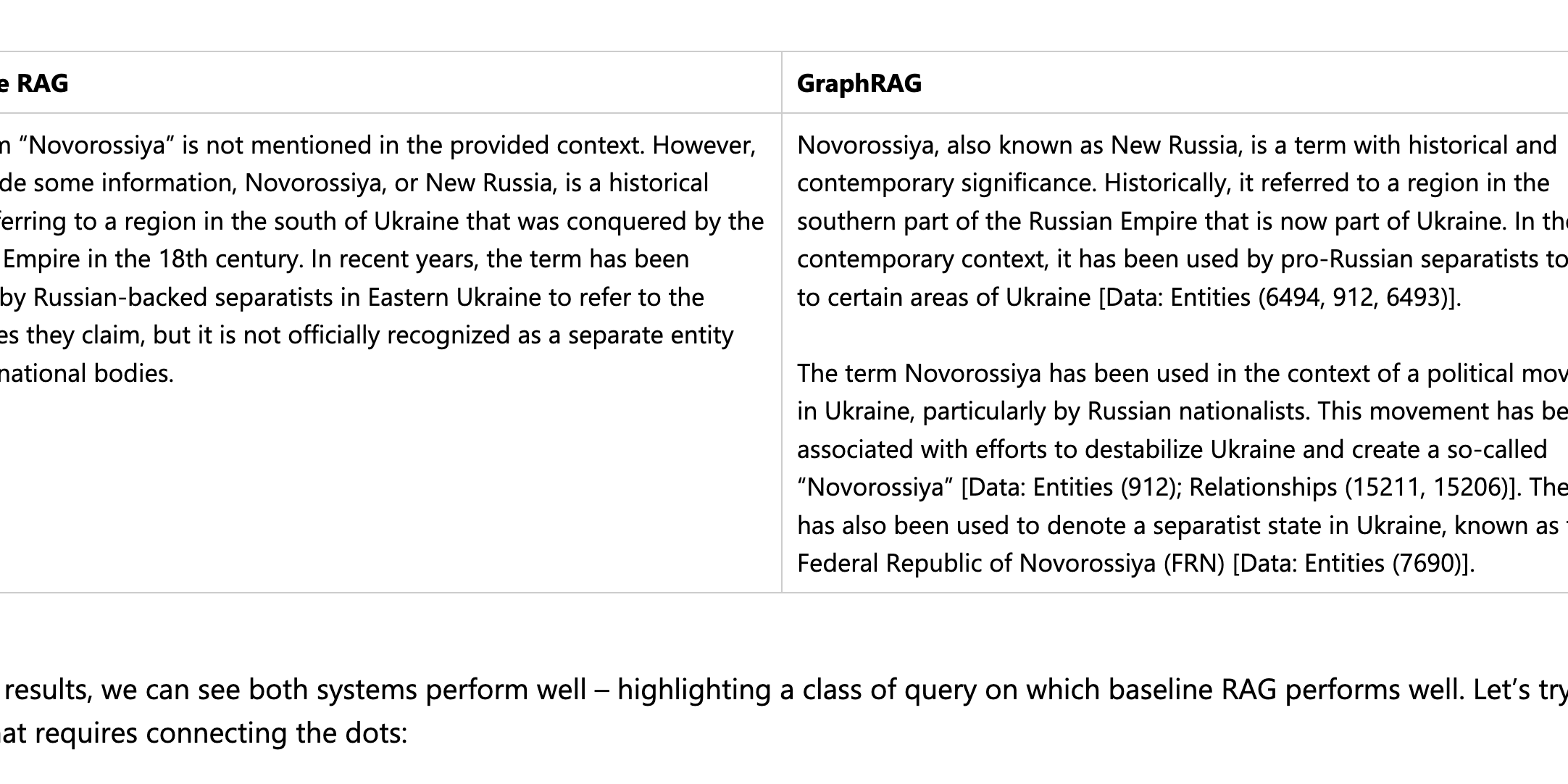
Unlocking New Insights in Private Data with GraphRAG: A Game-Changer for Finance
In a world awash with data, financial professionals constantly grapple with extracting actionable insights from proprietary datasets. Whether it's understanding market trends, tracking competitors, or identifying emerging risks, the challenge is turning disconnected data into a coherent narrative. This is where GraphRAG, a new Retrieval-Augmented Generation (RAG) technique introduced by Microsoft Research, is making waves.
GraphRAG combines the capabilities of large language models (LLMs) with graph machine learning to create knowledge graphs that structure, connect, and synthesize data from private datasets. It’s not just a technical leap—it’s a practical tool for industries like finance, where synthesizing complex, narrative-driven data can yield a competitive edge.
Why Finance Needs Better Tools for Private Data
Traditional RAG systems use vector similarity to retrieve relevant chunks of data, enabling LLMs to answer questions based on user queries. While effective for straightforward lookups, these systems struggle in scenarios that require:
Connecting the Dots: For questions that demand synthesizing insights across disparate data points, traditional RAG often falls short.
Holistic Analysis: Summarizing semantic concepts from vast datasets or lengthy documents can overwhelm standard approaches.
In finance, these shortcomings are evident when analyzing competitive landscapes or mapping related entities across fragmented datasets, such as market reports, earnings transcripts, and proprietary research.
GraphRAG: A Smarter Way to Work with Private Data
GraphRAG builds on baseline RAG by creating LLM-generated knowledge graphs. These graphs map out entities, relationships, and semantic clusters within the dataset, enabling the LLM to retrieve and synthesize relevant information more effectively.
Key Innovations:
- Entity Relationships: GraphRAG doesn’t just retrieve data—it understands the connections between entities, making it possible to answer complex queries like, “What are the emerging risks for Company X based on recent filings?”
- Whole-Set Reasoning: By clustering the dataset into thematic groups, GraphRAG can summarize key insights, such as the top five trends in quarterly earnings reports.
- Provenance: Each insight is grounded in the source material, ensuring transparency and enabling human verification.
Practical Applications in Finance
Here’s how GraphRAG could transform workflows in the financial industry:
Competitive Analysis: Identify competitors’ strategic moves by linking mentions across analyst calls, press releases, and industry news. For example, tracing a competitor’s expansion strategy from scattered references in different documents becomes seamless.
Product Mapping: Quickly build a landscape of competing products by analyzing regulatory filings, customer reviews, and sales reports.
Risk Assessment: Understand interconnected risks by aggregating data from fragmented sources like regulatory notices, ESG reports, and media articles.
The GraphRAG Advantage
In tests conducted by Microsoft Research, GraphRAG consistently outperformed baseline RAG in scenarios requiring deep narrative understanding and synthesis. Consider the example shared in Microsoft’s blog:
- Query: "What has Novorossiya done?"
- Baseline RAG: Fails to connect the dots, retrieving irrelevant chunks.
- GraphRAG: Produces a detailed response grounded in source material, linking specific entities and their activities.
For finance professionals, this difference could mean faster, more reliable insights in time-sensitive situations like deal-making or crisis management.
Looking Ahead
As financial datasets grow in size and complexity, tools like GraphRAG will be essential for staying competitive. The combination of LLMs, knowledge graphs, and graph machine learning opens the door to better private dataset discovery, making it easier to uncover relationships, trends, and actionable insights.
At AnalystAI, we’re already leveraging similar approaches to help clients navigate their data, whether it's mapping out competitors, identifying relevant products, or synthesizing trends. As tools like GraphRAG evolve, the potential applications in finance and beyond will only expand.
For more details on Microsoft’s research, check out their blog on GraphRAG.