Why ChatGPT Alone Isn’t Enough
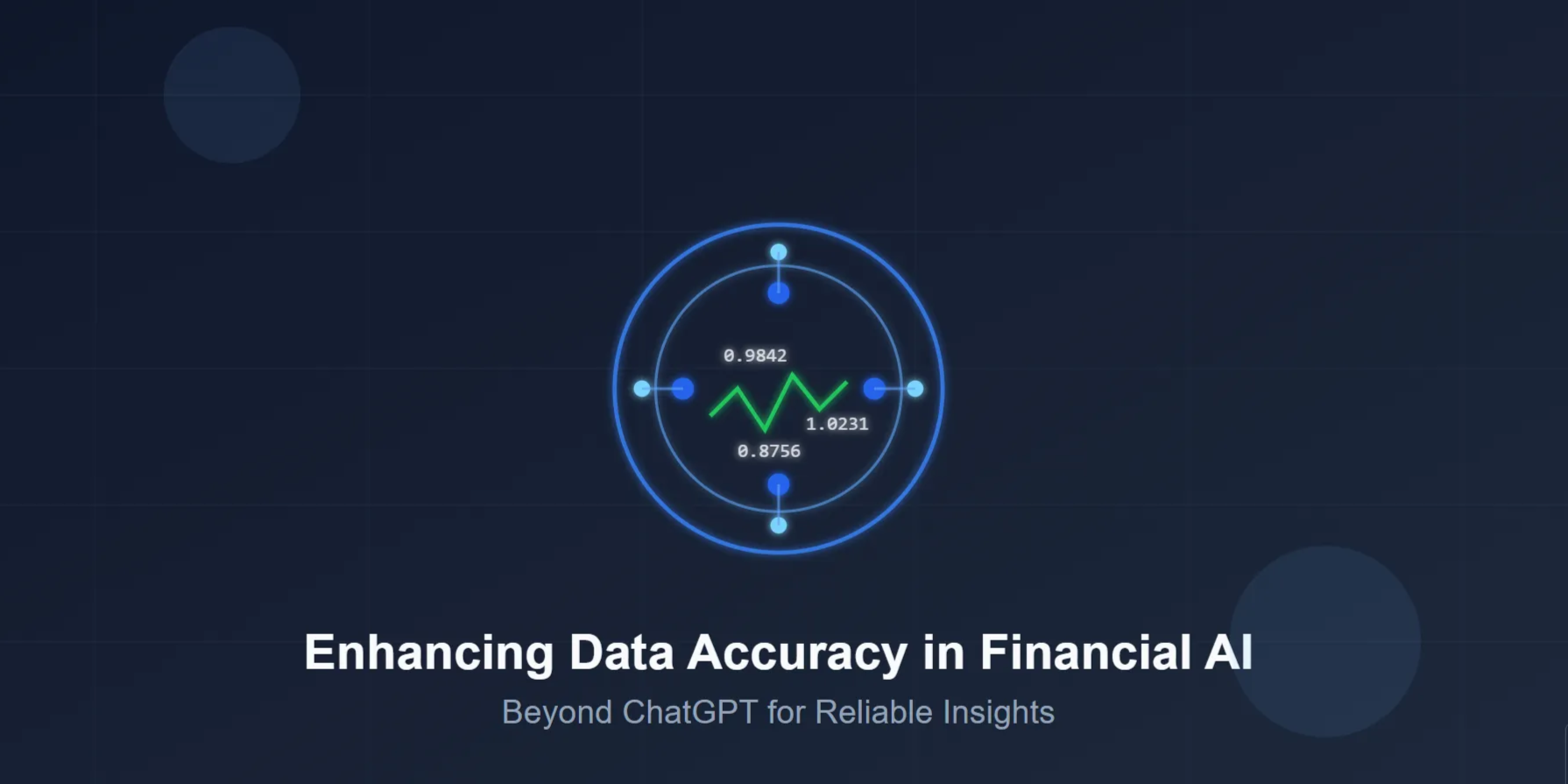
Beyond ChatGPT for Reliable Insights
Your firm might have already purchased ChatGPT enterprise. The issue is, however, it will pull random sources from the web, which you do not control, and you need to nudge it 100x to get it to a place where you like the output. Naturally this doesn’t scale, and the reason is that ChatGPT is not built for investors.
In fact, it’s a consumer product, and OpenAI has other priorities than focusing on finance use cases. Depending solely on OpenAI, ChatGPT means that you do not fully utilize the benefits of AI.
The Critical Role of Data Accuracy in Financial AI
You are building a financial model, there is a key driver, a data point that supports rest of the model and for some reason, it’s not cited. You forget in which document this was taken, and you have 1000s of PDFs on this market. You know that if you do not back up these numbers, that one IC member can ask a question and kill your deal.
Nobody wants that, but this is the case for many of us. Sometimes you are looking for data to see if your thesis is correct, so you look for it all accross web, PDFs, previous deal docs. This process takes time and having the wrong data point with no source can lead you into the wrong direction.
Single vs. Multiple Prompt Techniques: Impact on AI Outputs
How AI systems respond to input is crucial in financial contexts. A single broad question often yields generic answers, which may lack the detail necessary for financial analysis. For example, asking “What is the financial outlook for next quarter?” will return a general response and miss key insights.
In contrast, multiple, specific prompts lead to more refined results. For instance, breaking down the request into steps—analyzing market trends, considering historical data, and integrating these factors into a forecast—helps AI deliver more accurate and tailored insights. This method is particularly valuable in finance, where complex datasets require a step-by-step approach to ensure all relevant factors are considered.
The Importance of Well-Crafted Prompts in AI Systems
The structure of prompts can significantly impact AI performance in finance. Inadequate or overly broad prompts may lead to superficial or misleading conclusions. For example, asking AI to "analyze market trends and provide investment recommendations" without context might result in generic advice.
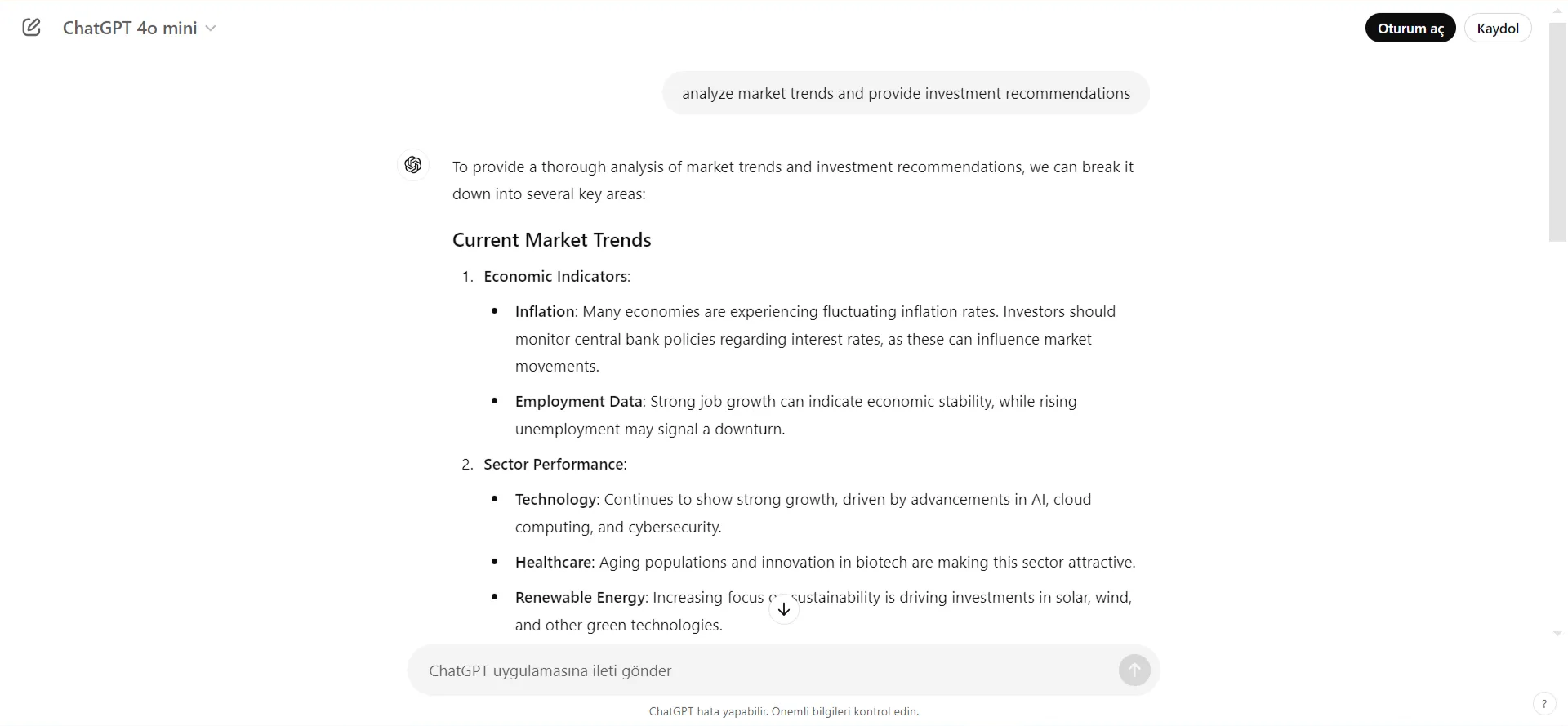
a screenshot of a basic GPT-4 prompt on market trends and investment recommendations
However, a precisely worded prompt—such as requesting an analysis of market trends specific to the tech sector, factoring in interest rate changes—can yield highly relevant and precise results.
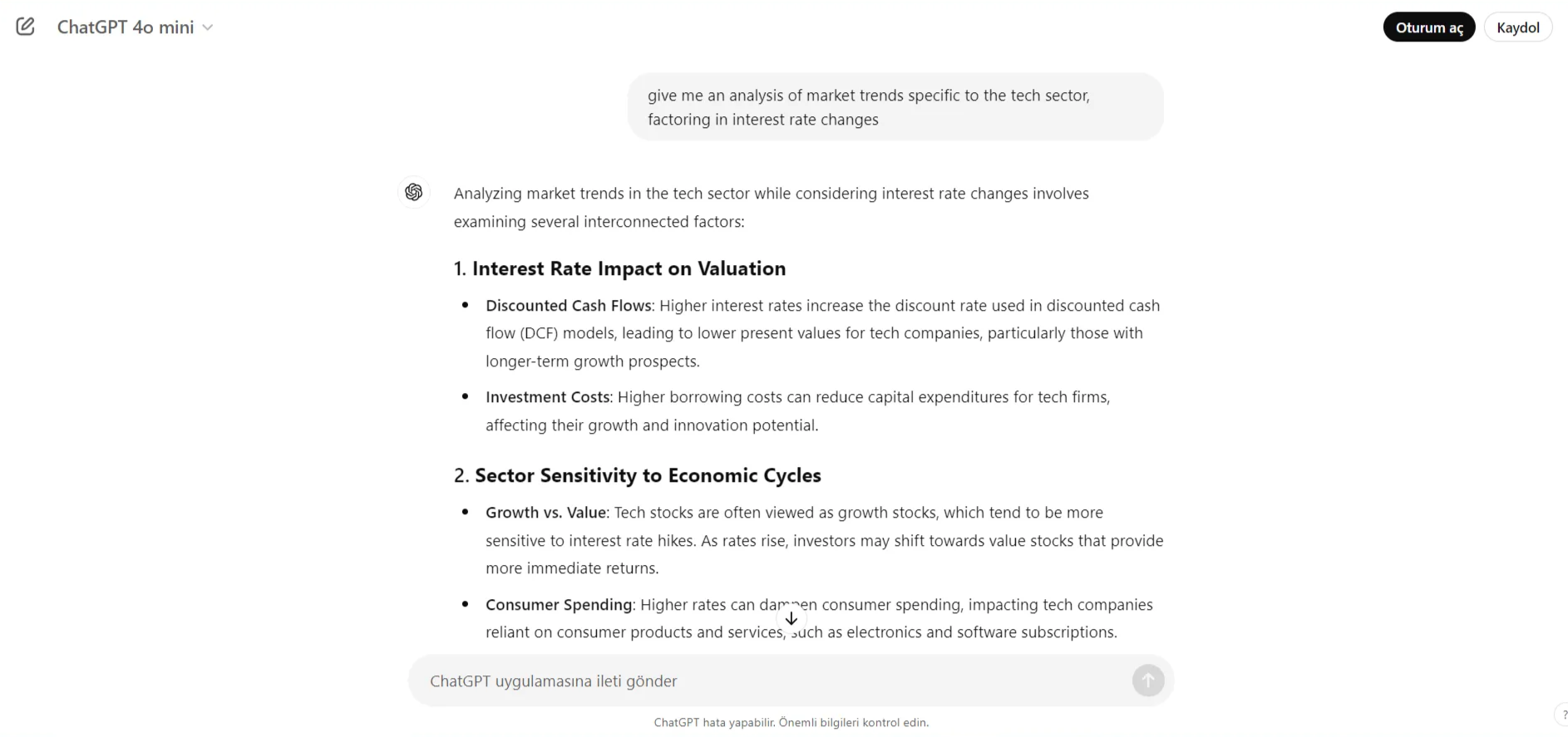
a screenshot of a contextual ChatGPT prompt on market trends specific to the tech sector
Why ChatGPT Alone Isn’t Enough for Financial Data Accuracy
Despite its capabilities, ChatGPT operates on probabilistic models and may produce outputs based on likelihood rather than certainty. In finance, where even small errors have large consequences, this lack of deterministic accuracy is a concern. A report generated by ChatGPT might sound plausible, but without rigorous validation, could contain inaccuracies that lead to faulty decisions.
Financial analysis often involves interpreting nuanced data, understanding regulatory frameworks, and making complex predictions. While ChatGPT can support some of these tasks, relying on it alone may not provide the precision necessary for high-stakes financial environments.
Advanced Techniques for Improving AI-Driven Financial Data Quality
To maintain high standards of accuracy, financial institutions should adopt several advanced strategies:
- Contextual Calibration: Tailor models to specific financial contexts to improve their accuracy. This involves fine-tuning AI models with industry-specific data, ensuring they understand the unique language and nuances of financial datasets.
- Multi-Step Validation: Implement a multi-layered validation process to cross-check AI-generated data against external sources or historical data. This ensures that forecasts and insights are validated and reliable.
- Feedback Loops: Set up feedback loops to continually refine AI models based on real-world outcomes. This ongoing refinement process improves the system’s accuracy over time.
- Hybrid Models: Combine different AI models to leverage the strengths of each. Pairing a generative model with a specialized financial prediction tool can result in more nuanced and accurate insights.
- Regular Audits: Periodic reviews of AI systems and data accuracy help ensure that models remain effective and adapt to evolving market conditions.
Conclusion
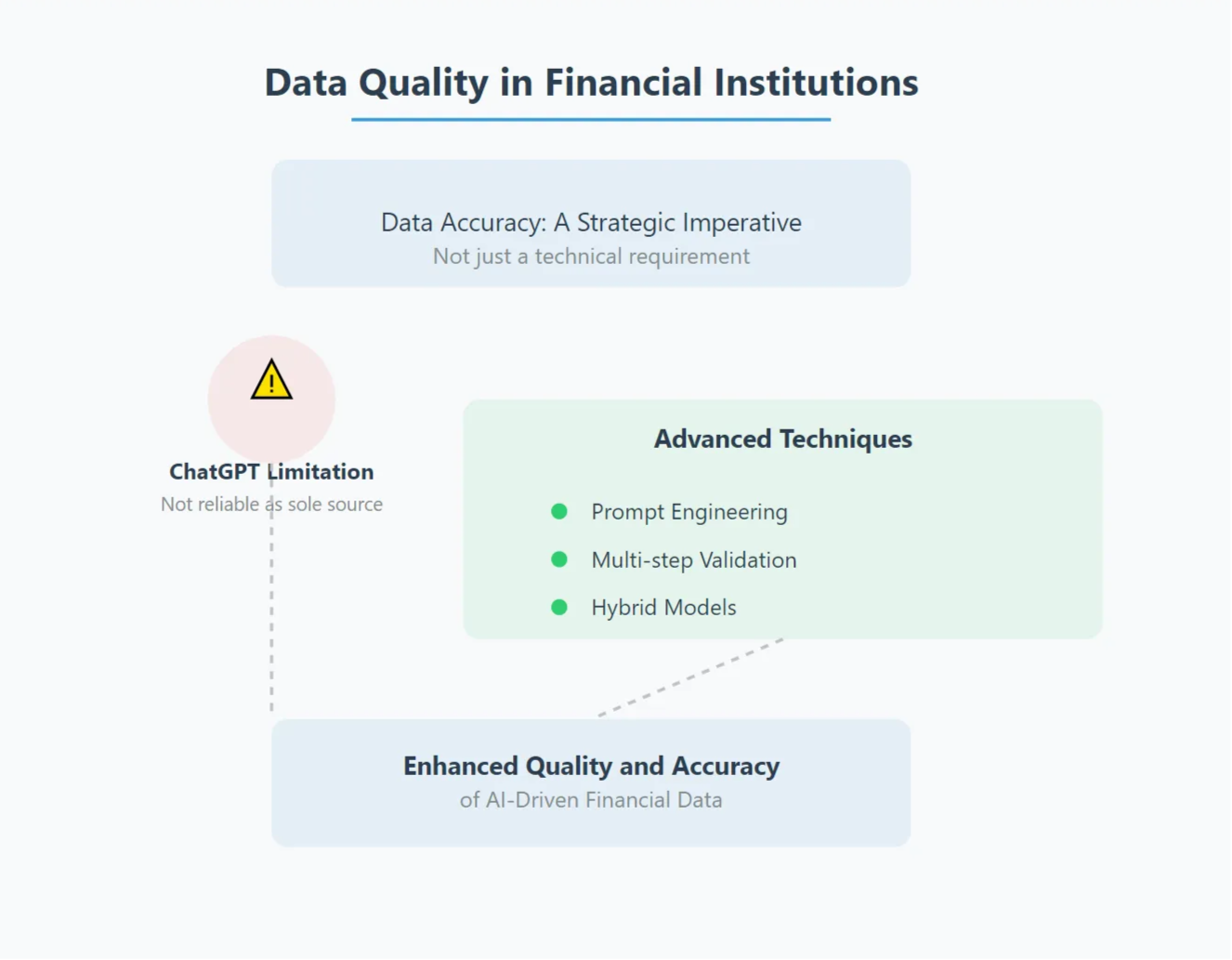
an infographic illustrating data quality in financial institutions
For financial institutions, data accuracy is more than a technical concern—it's a strategic imperative. While tools like ChatGPT offer valuable capabilities, they should not be relied upon as the sole source of financial insights. By adopting advanced techniques such as prompt engineering, multi-step validation, and hybrid models, financial institutions can significantly enhance the quality and accuracy of AI-driven data.
Investing in these advanced methods will allow financial institutions to maintain their competitive edge, making better decisions and providing greater value to their clients.